By Neil Scrimgeour, Kieran Hirlam, Eric Wilkes and Mango Parker, The Australian Wine Research Institute
Australian Wine Research Institute researchers have tested the use of mid-infra-red spectroscopy as a rapid screening tool for likely smoke exposure to grapes and wine.
The incidence of uncontrolled bushfires is increasing worldwide. As a result, the risk to grapevines from smoke exposure is an issue that the wine industry is progressively being forced to address. When grapes are exposed to smoke it can result in wines with undesirable sensory characteristics, such as ‘smoky’, ‘burnt’, ‘ash tray’ or ‘medicinal’, depending on the smoke composition and the length of smoke exposure.
The compounds in smoke primarily responsible for these attributes are the volatile phenols produced when wood is burnt.
These compounds can be absorbed directly by grapes, leaves and stems and can bind to sugar compounds in grapes to produce glycosides that have no smoky aroma. During fermentation (and over time in barrel or bottle) these glycosides can break apart, releasing the volatile phenols into the wine.
This release of volatile phenols can also occur in the mouth via the action of salivary enzymes, further contributing to the perception of smoke characters during tasting.
Testing grapes or wine for evidence of smoke exposure involves the use of sophisticated and expensive analytical instrumentation to separately determine the concentration of individual volatile phenols and glycosides. Interpretation of results requires comparison with background levels of these compounds in non-smoke-exposed grapes.
The analytical methods require a relatively high level of technical expertise to administer and cannot be applied in real time to understand the potential risk posed by smoke exposure.
A rapid screening test that could be used to determine the extent of impact from smoke exposure would be extremely valuable for the wine industry.
Developing a rapid screening method for grapes and wine
Mid-infra-red (MIR) spectroscopy is an analytical method with broad application for the measurement of chemical attributes in grapes, juice and wine, with testing typically taking less than a minute to complete.
It was therefore considered a good candidate for rapid assessment of smoke-exposed grapes and wine. To assess the viability of using MIR spectroscopy for this application, a series of grape and wine samples were collected during the 2020 vintage, with some expected to be at high risk of impacts from smoke exposure and others perceived to be at lower risk.
Each sample was assessed using both MIR spectroscopy and conventional chemical analysis (GC-MS and LC-MS) for free volatile phenols and glycosides.
In total, 349 grape samples and 388 wine samples were screened, with statistical analysis carried out using a number of different cloud-based chemometrics platforms.
Two approaches were used for the data analysis:
- Development of linear regression models using quantitative data from the chemical analysis; and
- Development of classification models using a nominal smoke risk rating of ‘low’, ‘medium’ or ‘high’ to define the extent of the impact of exposure.
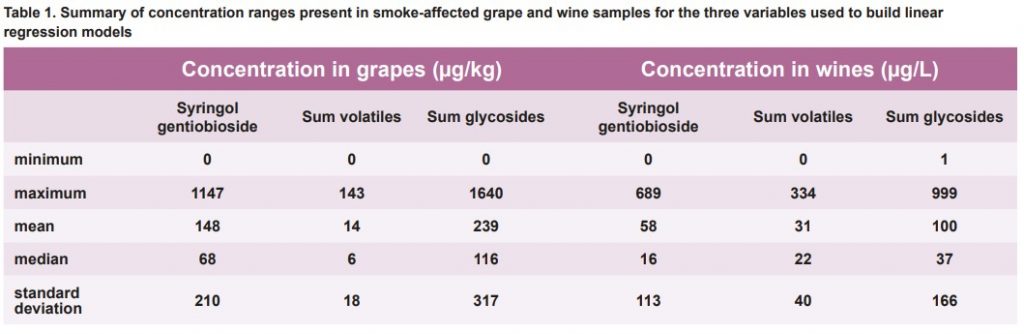
In each case, the data was randomly split to assign 70% of the samples to a training set, used for model building purposes, and an independent holdover set comprising 30% of the samples, used for model validation.
Generally speaking, the absolute concentrations of individual smoke marker compounds in smoke-affected grapes and wines are extremely low compared to those of other grape and wine analytes. The sums of the concentrations for volatile phenols and glycosides were therefore chosen to model the impacts of smoke exposure.
The concentration of syringol gentiobioside was also considered a viable option as this was the predominant smoke marker compound present in most of the grape and wine samples screened from vintage 2020.
Accordingly, linear regression models were built using three different output variables:
- sum of volatile phenol concentrations
- sum of glycoside concentrations
- Concentration of syringol gentiobioside Summary statistics for these three variables are provided in Table 1.
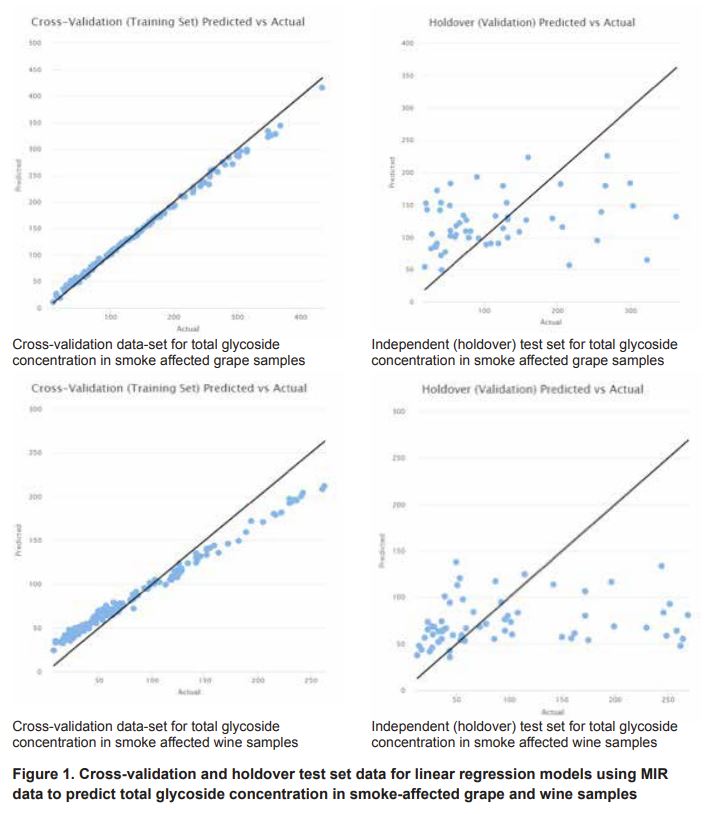
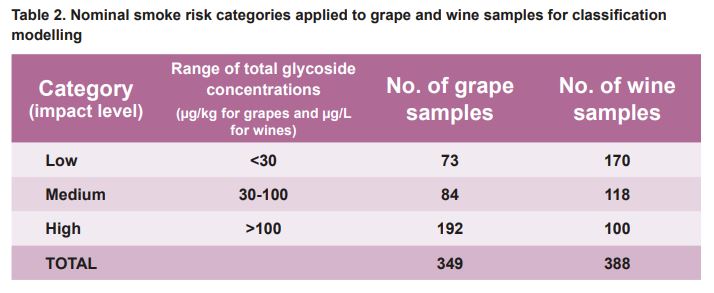
Results from the linear regression models
Results generated when applying linear regression models to the sum of glycosides in grapes and wine are shown in Figure 1.
None of the spectral models built allowed any of the three variables to be quantified with a reasonable degree of accuracy in either grape or wine samples.
Although the cross validation models appeared to show promise for quantifying the extent of smoke impact, the prediction error with independent (holdover) test samples was very high, as indicated by the extremely poor correlation between actual and predicted values for the holdover set.
Developing classification models
An alternative approach considered was the development of classification models, based on the assignment of a nominal smoke risk rating of ’low’, ‘medium’ or ‘high’, using the same three analytical variables as those used for the linear regression models.
Of these, the most successful models were based on the total glycoside concentration in the grape and wine samples.
The range of concentration values assigned to the individual risk categories and the number of grape and wine samples that fell into each category are summarised in Table 2.
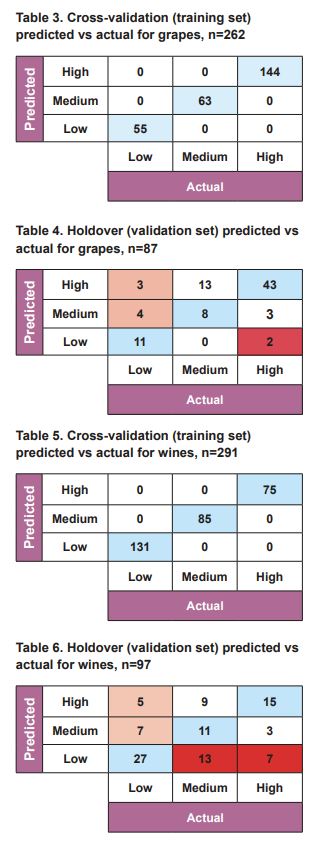
For grape samples, the most promising classification prediction was built using a Gradient Boosting Machine Learning model, which produced a cross-validation accuracy of 100% (correct sample classification with the training set, Table 3).
The independent validation set showed a prediction accuracy of 71% (Table 4).
The rate of false positive predictions (samples predicted as being medium or high risk, but actually in the low category) was 8% (7 samples out of 87, highlighted in orange in Table 4).
The rate of false negative predictions (samples predicted as low but actually in the medium or high categories) was only 2% (2 samples out of 87, highlighted in red in Table 4).
Re-modelling was carried out using a reduced sample set, with a significant number of high category samples removed to provide a more balanced model (n = 165 [training set] and n = 54 [validation set]).
In this case, a Distributed Random Forest type model was developed and this exhibited a 17% false positive prediction rate (9 samples out of 54) for the validation samples and, importantly, a 0% false negative prediction rate (0 samples out of 54).
For wine samples, a Gradient Boosting Machine Learning classification model was again the most promising, with a cross-validation accuracy of 100% (Table 5) and an independent validation prediction accuracy of 55%.
The rate of false positive predictions (samples predicted as being medium or high, but actually in the low category) was 12% (12 samples out of 97, highlighted in orange in Table 6).
The rate of false negative predictions (samples predicted as low but actually in the medium or high categories) was 21% (20 samples out of 97, highlighted in red in Table 6).
Again, re-modelling was carried out using a reduced sample set, with a significant number of high category wine samples removed to provide a more balanced model (n = 225 [training set] and n = 75 [validation set]).
The revised Gradient Boosting Machine Learning model exhibited a 13% false positive prediction rate (10 samples out of 75) for the validation samples and a 3% false negative prediction rate (2 samples out of 75).
Summary
The use of MIR spectral data to classify potentially smoke-affected grapes as low, medium or high risk using classification models shows significant promise as a rapid screening tool for likely smoke exposure.
However, further work is required to better understand the compounds that contribute to false negative results. To date, it appears that linear regression models used to quantify the concentration levels of smoke marker compounds in grapes and wine do not have the required precision to be used as a meaningful screening tool.
Once refined, classification models such as these could be used for preliminary screening of grape and wine samples potentially affected by smoke.
Those samples classified as being ‘medium’ or ‘high’ risk could then be subjected to more detailed chemical analysis using existing GC-MS and LC-MS techniques.
Those samples classified as ‘low’ risk may not require further chemical analysis, especially if the potential risk of returning false negative results from the initial screening procedure can be eliminated.
Further development work is required to assess whether moving from a 3-class model to a 2-class model (samples being categorised as either low or high) or adjustment of the low smoke impact threshold (currently set at 30µg/kg for the sum of glycosides in grapes and 30µg/L for the sum of glycosides in wines) will have beneficial impact on the prediction accuracy of the classification models.
The classification models built for wine samples showed less promise, although a 3% false negative prediction level was achieved using a reduced and more balanced sample set.
This may be due to the relatively low concentrations of glycosides present in smoke-affected wines, compared with grapes, and the higher levels of volatile phenols present in the wine samples.
It is also likely that other phenolic and glycosidically bound compounds that naturally occur in wines could generate interference in the spectral data and affect the performance of the models.
Several parallel (unpublished) studies of smoke taint screening have suggested that other analytical methods may have some utility in the detection of smoke-related compounds in grapes and wines, although none as yet appear to provide a robust solution for rapid screening.
Ultimately, a combination of methods may be required to provide a high degree of confidence for preliminary screening of samples.
Acknowledgements
The AWRI’s communications are supported by Australia’s grapegrowers and winemakers through their investment body Wine Australia, with matching funds from the Australian Government.
The AWRI is a member of the Wine Innovation Cluster in Adelaide. The AWRI gratefully acknowledges Yoko Obara, Yoshiko Takahashi and their contacts in Tokyo, Nagoya and Kanazawa, who between them raised and donated funds for smoke taint research by staging tastings featuring Australian wine.
Reference
Herderich M.J. and Krstic, M.P. (2020) Mitigation of climate change impacts on the national wine industry by reduction in losses from controlled burns and wildfires and improvement in public land management. Available from: https://www.wineaustralia.com/research/projects/mitigation-of-climate-change-impacts
This article was originally published in the Autumn 2021 issue of Wine & Viticulture Journal. To find out more about our quarterly magazine, or to subscribe, click here!
Are you a Daily Wine News subscriber? If not, click here to join our mailing list. It’s free!